
本記事はソラコムが提供する「SORACOM公式ブログ」に掲載された「AI とクラウド型カメラを組み合わせた安心見守りシステム」を再編集したものです。
目次
問題が起きた時だけ通知を受けたい人が倒れたことをAIを使って認識できるようにコンピュタービジョンモデルを開発する
誰かが倒れた時にライブ通知してくれるようにする
まとめ
Receiving Notifications During Emergencies
Developing a Computer Vision Model for Fall Detection
Implementing a Live Notification Feature
皆さん、こんにちは。ソラコムでソフトウェア開発のインターンをしています、伊佐山(ニックネーム: sean)です。
アメリカの大学生として、東京でのインターンシップ中、祖父母の家に滞在できることはとても幸運です。ただ、仕事に出かける際には常に心配事があります。例えば、「家で何かが起きた場合どうしよう?」「緊急事態が発生したらどう対応する?」「もし事故があった場合、どうやって知ることができる?」などです。
祖父母のように二人暮らしをしていると、このような不安が日常的に生じることが少なくないと思います。
問題が起きた時だけ通知を受けたい
ソラコムのクラウド型カメラサービス「ソラカメ」は、カメラの管理を効率化する革新的な方法です。ソラカメを使えば従来の常時確認ではなく、イベントベースのアプローチが可能です。
従来は、人間がカメラ映像を常に確認し、問題が発生した場合に対応する必要がありました。しかしこの場合、仕事や授業中といった場合には確認し続けることはできません。また、AIを常時稼働させるのもリソースとコストがかかります。一方ソラカメは、カメラ(例えばATOM Cam 2)が自律的にイベントを検出し、その情報をクラウドに送信することができる「クラウドモーション検知”無制限”録画機能」があります。これにより、必要な場合にのみAIプログラムを起動させることができ、リソースの使用を最適化しコストを削減できます。
クラウドモーション検知”無制限”録画機能を利用するには、「クラウド常時録画ライセンス」または、「クラウドモーション検知”無制限”録画ライセンス」が必要です。するとソラカメ対応カメラがモーションまたはサウンド検知した際の映像のみがクラウドにアップロードされ保存されます。ここから保存された録画データを自分のシステムと連携するためにソラカメAPI を使用します。
このようなイベントベースのアーキテクチャは、効率的な確認方法を実現するだけでなく、運用コストや人的リソースの負担を軽減する点で非常に有効です。これにより、安全性やセキュリティの向上が期待できます。ソラコムのクラウドカメラサービスを導入することで、自宅やオフィスなどさまざまな環境で、効率的で安全な状況把握システムを構築することが可能です。
このブログでは、ソラカメ対応製品のATOM Cam 2を使用します。
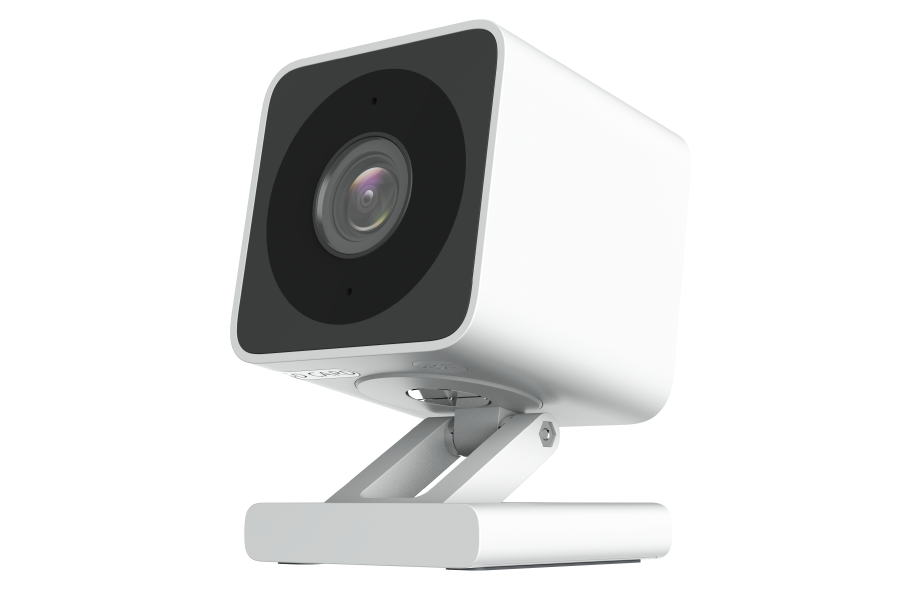
人が倒れたことをAIを使って認識できるようにコンピュタービジョンモデルを開発する
人間にとって「誰がいつ倒れ、いつ立ち上がったのか」を認識するのはとても簡単です。ですが、人間ではないカメラデバイスにはこのような分類問題に対処するためには機械学習(マシンラーニング)モデル、もっと詳しくはコンピュタービジョンモデルが不可欠です。具体的には、「倒れているか、倒れていないか」という分類問題に答えるために、YOLOv8とRoboflowを使用してコンピュタービジョンモデルを開発します。
YOLOv8は、YOLO(You Only Look Once)オブジェクト検出モデルの特定のバージョンを指します。YOLOv8は、スピードと正確さで知られるリアルタイム物体検出モデルの人気シリーズです。一方、Roboflowは、コンピュタービジョンプロジェクトのデータセットを管理、ラベリング、増強するためのプラットフォームとツールキットであり、特に物体検出のようなコンピュータ・ビジョンのタスクに焦点を当てています。YOLOv8とRoboflowを組み合わせてどう機能しているかを確認するには、こちらのデモをお試しください。
実は「倒れているか、倒れていないか」の分類自体は、ChatGPT等のマルチモーダルLLM(生成AI)でも可能です。ChatGPTは汎用的な使い方には有用ですが、学習が必要なケースや、動画を対象とする場合は使い辛い点があります。例えば、動画を直接アップロードできないため、「人が倒れてからどれくらい時間が経過しているか」といった質問に答えるのは非常に難しいです。YOLOv8とRoboflowを使うことで、それらを解決します。
このページの情報を参考に、以下の手順で進めました。
- データの取得:ATOM Cam 2を使い、自分が部屋の中で動き回り、倒れた後に立ち上がるというシーンを捉えた約30秒の動画を撮影しました。この動画は、「立っている」、「倒れている」、「倒れた」という特定の時間帯が明確に区別されるように撮影しました。これにより、将来的にはこれらの行動状態に自動的にラベル付けを行うモデルのトレーニングが可能になります。
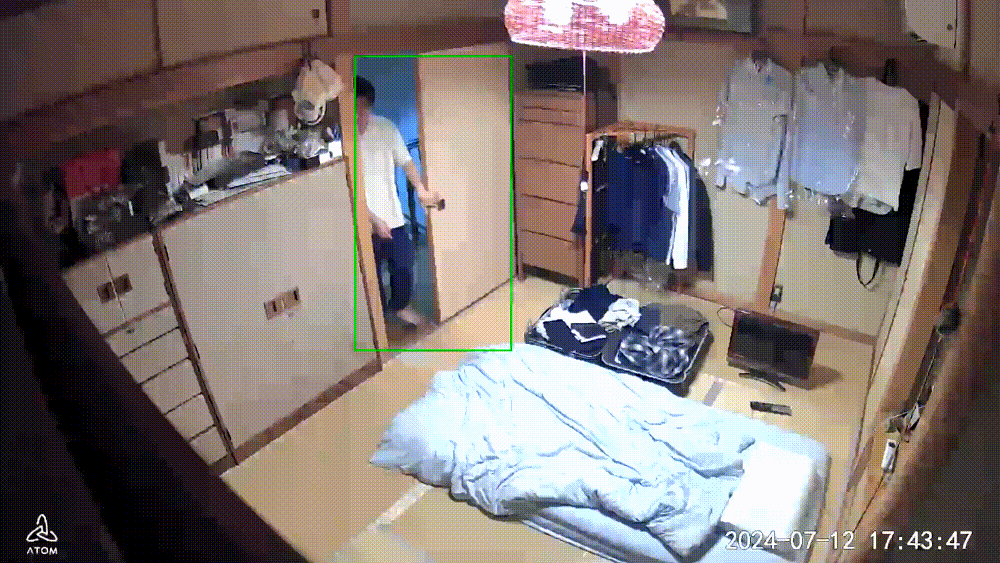
- フレームに分割:得られたmp4動画から、特定のフレームレート(fps)でフレームを抽出し、それぞれのフレームを静止画像として保存しました。収集した動画では、Roboflowで8fps、約250枚のフレームを作成しました。これらのフレームは、後でコンピュタービジョンモデルのトレーニングや分析に使用します。
- 「Standing」「Falling」「Fallen」のラベルを貼る:Roboflow のアノテーションツールを使い、フレームごとに手動でラベルを付けました。「立っている」なら「Standing」のラベル、「倒れている」なら「Falling」のラベル、「倒れた」なら「Fallen」のラベルを付けました。これでデータセットが完成です。
- モデルトレーニング:YOLOv8 を使ってモデルをトレーニングします。つまり、人間が立っている時、倒れている時、倒れた時、のパターンを認識できるようにモデルを「学習」させます。この場合、フレームの一つ一つが分類問題になります。学習するためには、モデルはデータからパターンを学習し、誤差を最小化するように調整されます。そのため、データが多いほど正確になります。
- モデルテスト:トレーニング完了後(約二時間)、開発したモデルを自由にテストできます。Roboflowの「Visualize」ツールでドラッグアンドドロップまたはアップロードを通じて、他の動画に対しても簡単に学習されたラベルを適用することができます。ラベルの隣にある数値はラベルの正しさの confidence(自信度)です。
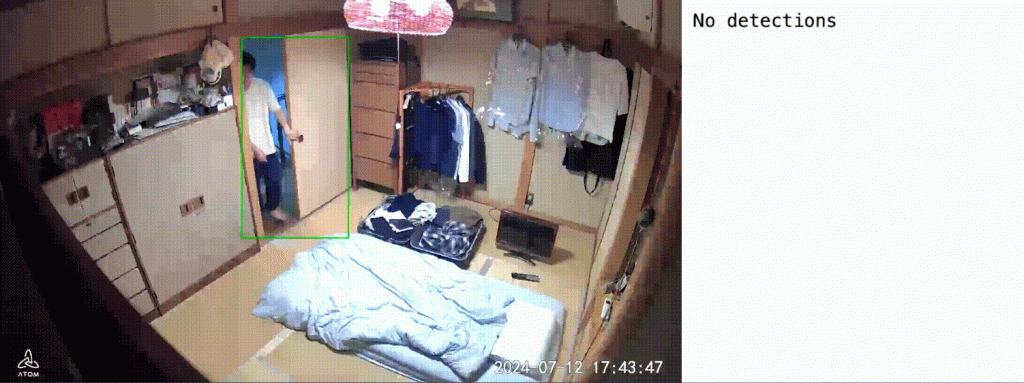
これでモデルのトレーニングとテストが完了しました。モデルのラベリング精度に満足できたら、いつでもデプロイできます。もし満足していない点がある場合は、データ量を増やしたり、別のモデルを使用してトレーニングを行うことをお勧めします。
誰かが倒れた時にライブ通知してくれるようにする
モデルを作成したので、次はライブ通知機能に取り組みます。これを実現するには色々な手段があります。その一つの全体構成がこちらです:
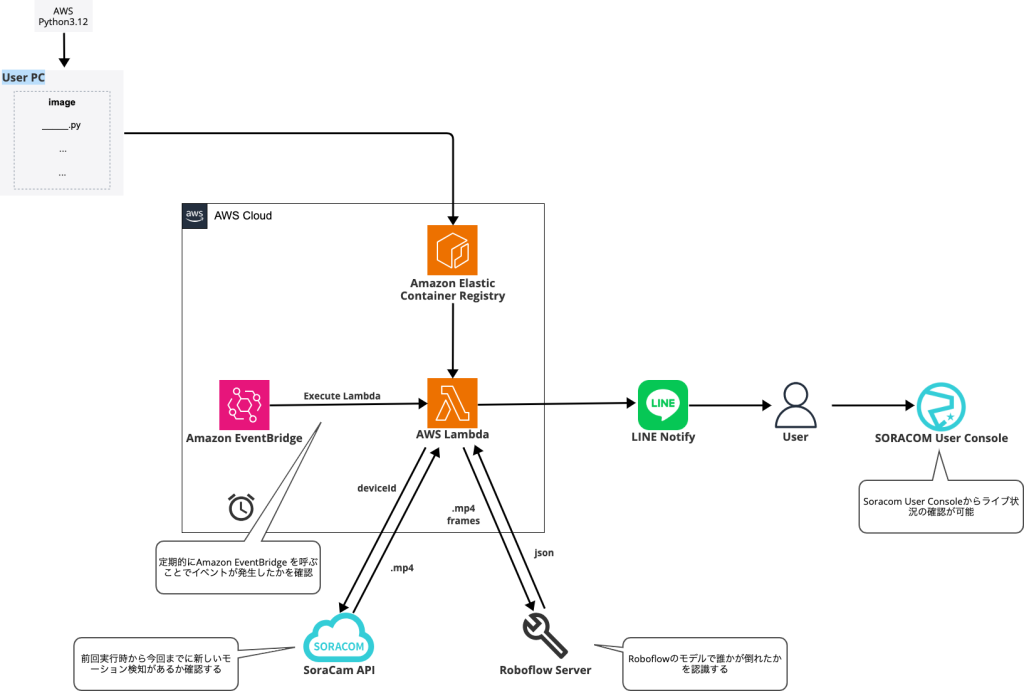
- Amazon EventBridge を使い定期的に AWS Lambda を呼び出します。これで新しいイベントが発生した時に確認できます。AWS Lambda では、以下の 2 から 4 までを実施します。
- ソラカメ (SoraCam) API で新しいイベントが発生したことを確認するのに必要なソラカメAPIを呼びます。具体的には、以下の API を呼び出します。
- listSoraCamDeviceEventsForDevice APIで新しいイベントを確認します。期間を絞ってイベント一覧を取得し、期間内にイベントを検知していたらエクスポートします。
- exportSoraCamDeviceRecordedVideo APIで、クラウドに保存された録画映像をダウンロードできる方式 (mp4 ファイルを zip 形式で圧縮したファイル) でエクスポートする処理を開始します。レスポンスにexportIdが返されるので、以下のAPIで取得します。この処理には時間が掛かるため、しばらく時間をあける必要があります。
- getSoraCamDeviceExportedVideo APIで、上記で取得したexportIdを指定すると、エクスポートが完了していたらファイルをダウンロードするためのURLを取得します。完了したかどうかは、statusプロパティで確認できます。completedでない場合は、しばらくしてからもう一度この API を呼び出してください。
- Roboflowのライブラリを用い、動画を分割してそれぞれ分類します。最初は Python 3.12 のランタイムを使って動かそうとしたのですが、NumPy が C++ で書かれていることから環境依存でうまく動かなかった点や、Roboflow のライブラリのサイズが大きすぎて AWS Lambda の Layer が使えなかった点から、コンテナイメージを使うようにしました。コンテナイメージの管理は、Amazon Elastic Container Registry(ECR) を利用しました。Roboflow のライブラリを用いて結果を取得したら、FALLEN が数回程度であれば「大丈夫」と判断し、FALLEN が続くと「対応が必要」と判断するロジックを加えます。
- FALLENが続いた場合、LINE に通知します。手順はこちらのレシピを参照してください。
- 必要に応じて、ユーザーコンソールでライブの状況を確認することができます。これにより、人が倒れたことを LINE で受け取ったあと、ライブ映像を簡単に確認できます。
これで AI を使ったクラウド型カメラの安心見守りシステムが完成です!
まとめ
プログラミングの経験がなくても、Roboflowはとても使いやすいツールなので、誰でも簡単にモデルを開発できます。自身のデータを使いモデルを開発することで、特定のユースケースに対する精度を最大限に引き出すことができます。そしてソラカメと組み合わせることで、ベビーモニターやペットカメラなどのアプリケーションも簡単に作成し、デプロイできます。もし自分でデータセットを作成したり、特定のニーズに応じたモデルの開発をしたい場合は、ぜひお試しください。
最近、AIサービスを使用したローコード IoT アプリケーションビルダー、SORACOM Flux が発表されました。特定の用途に特化した学習データは必要なく、かつ動画ではなく画像を評価できれば良い場合はSORACOM Flux を使用することで「誰かが倒れたかどうか」を簡単に検出できます。
参考:日本経済新聞の記事
SORACOM Fluxを使用する場合、GPT-4oなどのAI サービスを利用するため、画像についての分類問題に答えるプロセスははるかに簡単になります。SORACOM Flux は様々な AI モデルを利用でき、膨大なデータセットで学習・チューニングされているため、非常に幅広いケースに有効です。さらに SORACOM Flux のワークフロー設計ツールを使えば、倒れた後の「負傷しているか」「手当てが必要か」などのフォローアップの質問にも答えることができます。詳しくはぜひこちらを参照してください。
今回の例であるYOLOv8とRoboflowの組み合わせは、手作業でモデルを作成することにより、独自のユースケースに対して高い精度で対応できる利点があります。特に、GPT-4oなどの汎用 AI サービスでは不足している動画解析も容易に行えることが挙げられます。ニーズに応じて、最適なツールを使用してお試しください。
― ソラコム ソフトウェア開発インターン 伊佐山 光 (sean)
英語訳
Safety Monitoring System Using Cloud-Based Cameras and AI
Hello everyone! I’m Hikaru Isayama (nickname: sean), a Software Developer Intern at SORACOM.
As a college student from the United States, I am very fortunate to be able to stay at my grandparents’ home in Tokyo for the duration of the internship. However, I find myself worrying about a few things when I leave for the office: What if an accident occurs at home? What if there’s an emergency? How will I be alerted if something goes wrong?
In a two-person household like my grandparents’, these concerns must be common whenever someone leaves the home.
Receiving Notifications During Emergencies
Soracom Cloud Camera Services “SoraCam” is an innovative way to streamline surveillance camera management. SoraCam offers an event-based approach which can be used for a SoraCam compatible device, which can be used instead of the traditional constant monitoring approach.
Constant monitoring of surveillance systems typically requires a human to continuously watch camera feeds and respond to any issues that arise. This approach can be impractical and difficult to maintain, especially during work or school when continuous monitoring is not feasible. An alternative option is to have an AI (Artificial Intelligence) system that is programmed to alert users to significant events or anomalies. However, this approach can be resource-intensive and costly, as it requires the AI to be operational around the clock.
Soracom Cloud Camera Services enables devices like the ATOM Cam 2 to autonomously detect events and send this information to the cloud. By integrating this service with AI, we can optimize resource use and reduce costs, as the AI models are triggered only when event footage is sent to the cloud, ensuring that resources are used more efficiently.
To use the cloud motion detection recording function, it will require a license with motion detection from the Soracom Cloud Camera Services page. The stored recordings can then be exported to your own system using the SoraCam API.
This event-based architecture is highly effective for efficient surveillance management, as well as for reducing operational costs and alleviating the burden on human resources. By leveraging Soracom Cloud Camera Services, it becomes possible to create efficient and secure surveillance systems suitable for various environments, including homes and offices.
For this blog, I decided to use the ATOM Cam 2, a SoraCam compatible product.
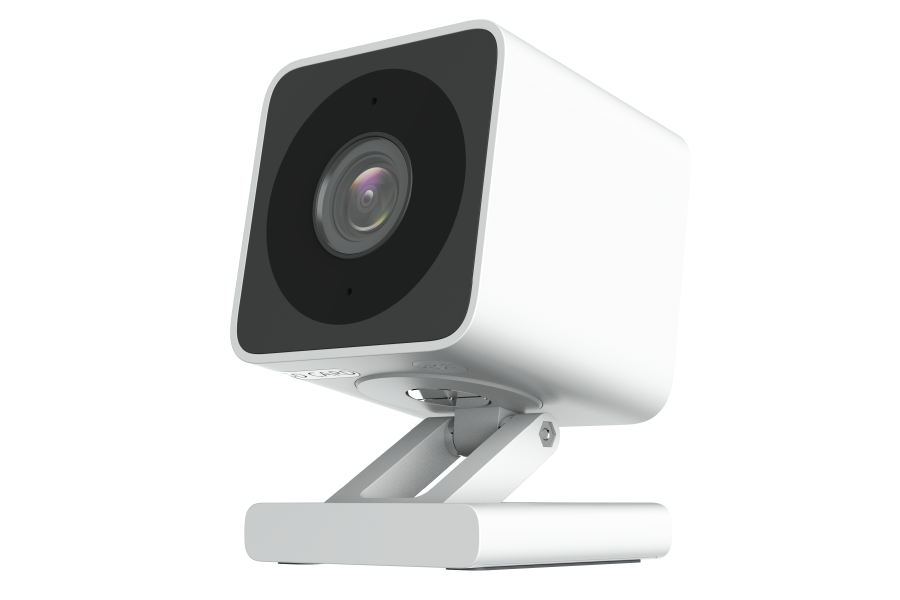
Developing a Computer Vision Model for Fall Detection
For us humans, it is very easy to recognize when a person is standing up, is falling down, or is fallen. But for machines and devices, machine learning models, more specifically computer vision models, are required to address such classification problems. Specifically, I used YOLOv8 and Roboflow to develop a machine learning model to be able to classify these states.
YOLOv8 refers to a specific version of the YOLO (You Only Look Once) object detection model. YOLOv8 is a popular series of real-time object detection models known for their speed and accuracy, which I will use to detect humans in the camera footage. Roboflow, on the other hand, is a platform and tool for managing, labeling, and augmenting datasets for machine learning projects, with a particular focus on computer vision tasks like object detection. To see how YOLOv8 and Roboflow work together, try the demo here.
Answering the question of whether a person has fallen or not is also possible with a multimodal LLM (generative AI) such as ChatGPT. However, while ChatGPT excels in general-purpose tasks, it struggles with specific use cases such as tasks that involve learning from or analyzing video files. For example, as it is not yet possible to upload video files to ChatGPT, it cannot analyze the duration a person has been on the ground from an MP4 file. To address these difficulties, I used YOLOv8 and Roboflow, which are better suited for handling video analysis and object detection tasks.
I referenced the steps and information on this page for the implementation of this model.
- Obtaining Data: Using the ATOM Cam 2, I collected approximately 30 seconds of video capturing myself moving around the room, falling down, and then getting up. This video was captured in such a way that specific time periods of “standing,” “falling,” and “falling down” were clearly distinguished. This will allow the model to be trained such that it will be able to label these periods with the videos provided during the testing phase.
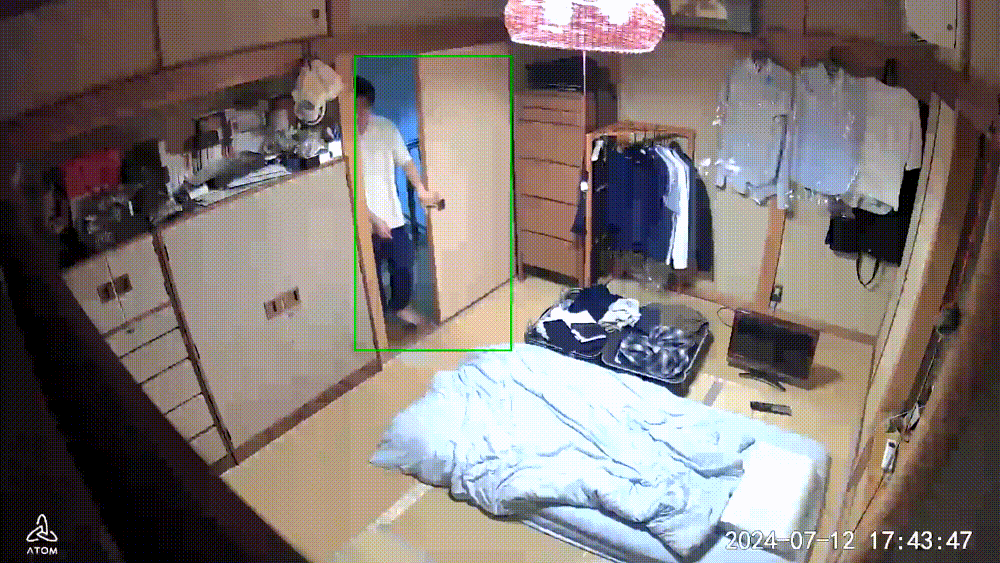
- Divide into Frames: From the resulting mp4 video, frames were extracted at a specific frame rate (fps) and each frame was saved as a still image. For this video, I used Roboflow to create around 250 frames at 8 fps.
- Label “standing,” “falling,” or “fallen”: For each frame, I manually labeled with the following states: “standing,” “falling,” and “fallen.” This will help the model “learn” when a person is standing, falling, or is fallen. With this, the dataset is ready to be trained on.
- Train Model using Roboflow: The model is trained using YOLOv8. That is, the model is “taught” to recognize patterns when a person is standing, falling, or has fallen in the video. In this case, each frame of the video becomes a classification problem. To learn, the model learns patterns from the data and is tuned to minimize errors. So the more data it has, the more accurate it will be.
- Model Testing: After training is complete (about two hours), the model is now ready to be tested using any video using Roboflow’s “Visualize” tool. Using this feature, videos can easily be drag-and-dropped or uploaded to freely test the model. The number next to the label is the confidence of the label’s correctness.
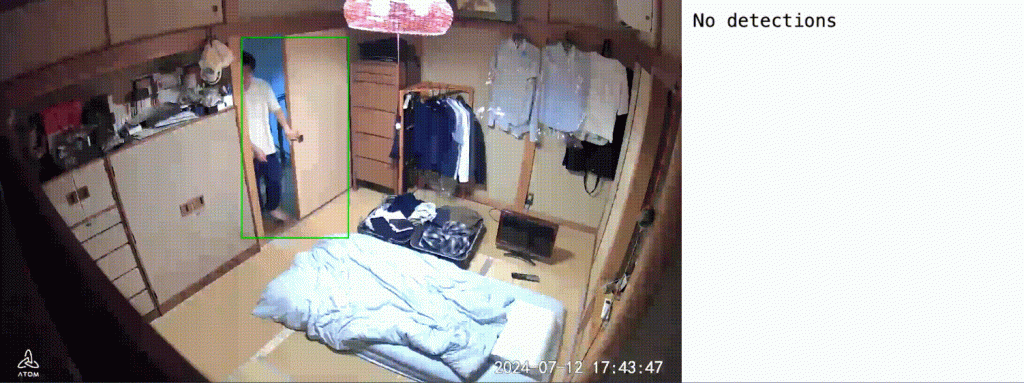
The model is now trained and tested, ready to be deployed at any time. In the case that you would like a model with better performance, some options are to increase the size of the dataset by importing more data, or training with a different model.
Implementing a Live Notification Feature
Now that the model has been developed, the next step is to implement the live notification feature. There are many ways to achieve this, but here is the overall structure of the one I will use:
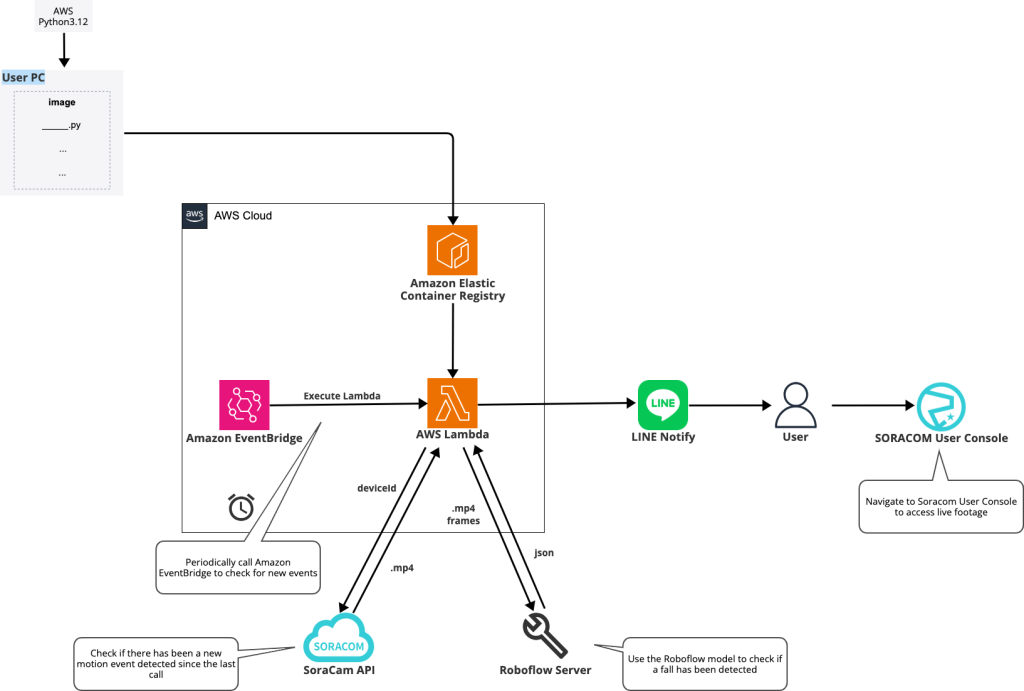
- Amazon EventBridge will be used to periodically call AWS Lambda. With this, we can check whether or not there is a new event captured live. AWS Lambda will then perform the steps 2 to 4 below.
- Using the SoraCam API, we will check if a new event has occurred. Specifically, we will call the following APIs:
- First, we will check for any new motion events using the listSoraCamDeviceEventsForDevice API. If there is an event within a set time before the current time, begin the export process.
- Using the exportSoraCamDeviceRecordedVideo API, we will retrieve the video from the cloud and begin the download process (mp4 file compressed in zip format). This will return the exportId, which is required in using the following API. *NOTE: even if the exportId is obtained, there is a chance that the export has not been initialized at that moment. Therefore, it may be necessary to add a time lag before finalizing the export
- Finally, use the exportId obtained above to use the getSoraCamDeviceExportedVideo API, retrieving a link to download the video from the cloud. It will also check if the export is completed or not with the status property; if it is not completed, call this API again after a few seconds.
- Using the Roboflow library, the videos are split and classified individually. At first, I attempted to run it using the Python 3.12 runtime; but since NumPy is written in C++, it was environment-dependent and did not work well. The size of the Roboflow library was also too large to use AWS Lambda’s Layer, so it was best to use container images, which were managed using Amazon Elastic Container Registry (ECR). Once the results were obtained using the Roboflow library, we implemented logic to classify how if the number of “fallen” tags was only a few it would not be classified as an emergency, and if the number of “fallen” tags continued, it would be classified as an emergency.
- In the case of an emergency, it would then notify the user through LINE. To deploy this model onto LINE, refer to this recipe.
- If necessary, the live status can be checked in the Soracom user console. This makes it easy to check the live video after receiving a message on LINE that a person has fallen.
With this, a safety monitoring system using cloud-based cameras and AI is complete!
Summary
Roboflow’s user-friendly interface allows users with minimal programming experience to easily create computer vision models. By leveraging their own data, users can also tailor models to achieve maximum accuracy for their specific needs. When combined with Soracom Cloud Camera Services, it makes building and deploying a variety of customized applications such as baby monitors or pet cameras very straightforward. For those interested in creating custom datasets or developing models tailored to specific needs, exploring Soracom Cloud Camera Services and Roboflow is a great next step.
Recently, SORACOM Flux, a low-code IoT application builder using AI services, was announced. If there is no requirement to train specific data, and only need to classify images rather than videos, SORACOM Flux provides an alternate and easy way to detect “if someone has fallen.”
Reference: Nihon Keizai Shimbun article
When using SORACOM Flux, the process of answering classification questions about images is much easier as it uses AI services such as GPT-4o. SORACOM Flux is available with a variety of AI models, trained and tuned on massive data sets, making it very effective for a wide range of cases. The SORACOM Flux workflow design tools also makes it possible to easily answer follow-up questions such as “is there an injury?” or “is there a need for medical attention?” after detecting a fall. For more information, read here:
Using YOLOv8 and Roboflow, I was able to manually create a model that can handle my unique use case with high accuracy. This model is also designed to handle video formats and live footage, making it easy to integrate into your own system. Depending on your needs and interests, feel free to choose the tool that best suits your project.
― Soracom Software Development Intern, Hikaru Isayama (sean)
投稿 AI とクラウド型カメラを組み合わせた安心見守りシステム は SORACOM公式ブログ に最初に表示されました。
この連載の記事
-
第466回
デジタル
カメラとAIで楽器演奏シーンを簡単に残す、IoTプロトタイピングの裏側 -
第465回
デジタル
数千を超えるIoT機器を管理!生成AIで設備運用を効率化するhacomonoの挑戦 -
第464回
デジタル
SORACOMのビジネスパートナープログラムに、新たに5社の認定済パートナーが参画、SORACOM Harvest Data で日時データをタイムスタンプとして利用可能に takuyaのほぼ週刊ソラコム 08/31-09/13 -
第463回
デジタル
SORACOM Lagoon 3 の Alert rule の考え方をマスターして、最適な通知を作成 -
第462回
デジタル
LTE USB ドングル UD-USC1 を SORACOM サービスと組み合わせて利用する -
第461回
デジタル
EV充電インフラを保護する5つの方法 -
第460回
デジタル
IoT プロジェクトの課題を解決する、SORACOM サービスの活用事例 -
第459回
デジタル
ATOM Cam 2 専用 LAN アダプターを販売開始、ソラカメのクラウド録画マルチストリーミング再生機能をリリース takuyaのほぼ週刊ソラコム 08/17-08/30 -
第458回
デジタル
IoTで接続された充電ステーションがEVの普及を促進する -
第457回
デジタル
SORACOM Napterがさらに便利に!リモートアクセスの安全性と利便性を両立したWebターミナルのご紹介